Topic:Chemical Reaction Prediction
What is Chemical Reaction Prediction? Chemical reaction prediction is the process of predicting the outcome of chemical reactions using machine learning models.
Papers and Code
Dec 30, 2024
Abstract:Understanding the long-time dynamics of complex physical processes depends on our ability to recognize patterns. To simplify the description of these processes, we often introduce a set of reaction coordinates, customarily referred to as collective variables (CVs). The quality of these CVs heavily impacts our comprehension of the dynamics, often influencing the estimates of thermodynamics and kinetics from atomistic simulations. Consequently, identifying CVs poses a fundamental challenge in chemical physics. Recently, significant progress was made by leveraging the predictive ability of unsupervised machine learning techniques to determine CVs. Many of these techniques require temporal information to learn slow CVs that correspond to the long timescale behavior of the studied process. Here, however, we specifically focus on techniques that can identify CVs corresponding to the slowest transitions between states without needing temporal trajectories as input, instead using the spatial characteristics of the data. We discuss the latest developments in this category of techniques and briefly discuss potential directions for thermodynamics-informed spatial learning of slow CVs.
Via
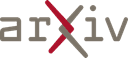
Dec 14, 2024
Abstract:Colloidal synthesis of nanocrystals usually includes complex chemical reactions and multi-step crystallization processes. Despite the great success in the past 30 years, it remains challenging to clarify the correlations between synthetic parameters of chemical reaction and physical properties of nanocrystals. Here, we developed a deep learning-based nanocrystal synthesis model that correlates synthetic parameters with the final size and shape of target nanocrystals, using a dataset of 3500 recipes covering 348 distinct nanocrystal compositions. The size and shape labels were obtained from transmission electron microscope images using a segmentation model trained with a semi-supervised algorithm on a dataset comprising 1.2 million nanocrystals. By applying the reaction intermediate-based data augmentation method and elaborated descriptors, the synthesis model was able to predict nanocrystal's size with a mean absolute error of 1.39 nm, while reaching an 89% average accuracy for shape classification. The synthesis model shows knowledge transfer capabilities across different nanocrystals with inputs of new recipes. With that, the influence of chemicals on the final size of nanocrystals was further evaluated, revealing the importance order of nanocrystal composition, precursor or ligand, and solvent. Overall, the deep learning-based nanocrystal synthesis model offers a powerful tool to expedite the development of high-quality nanocrystals.
Via
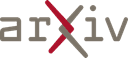
Dec 06, 2024
Abstract:Planning and conducting chemical syntheses remains a major bottleneck in the discovery of functional small molecules, and prevents fully leveraging generative AI for molecular inverse design. While early work has shown that ML-based retrosynthesis models can predict reasonable routes, their low accuracy for less frequent, yet important reactions has been pointed out. As multi-step search algorithms are limited to reactions suggested by the underlying model, the applicability of those tools is inherently constrained by the accuracy of retrosynthesis prediction. Inspired by how chemists use different strategies to ideate reactions, we propose Chimera: a framework for building highly accurate reaction models that combine predictions from diverse sources with complementary inductive biases using a learning-based ensembling strategy. We instantiate the framework with two newly developed models, which already by themselves achieve state of the art in their categories. Through experiments across several orders of magnitude in data scale and time-splits, we show Chimera outperforms all major models by a large margin, owing both to the good individual performance of its constituents, but also to the scalability of our ensembling strategy. Moreover, we find that PhD-level organic chemists prefer predictions from Chimera over baselines in terms of quality. Finally, we transfer the largest-scale checkpoint to an internal dataset from a major pharmaceutical company, showing robust generalization under distribution shift. With the new dimension that our framework unlocks, we anticipate further acceleration in the development of even more accurate models.
Via
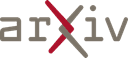
Dec 05, 2024
Abstract:Computing high dimensional potential surfaces for molecular and materials systems is considered to be a great challenge in computational chemistry with potential impact in a range of areas including fundamental prediction of reaction rates. In this paper we design and discuss an algorithm that has similarities to large language models in generative AI and natural language processing. Specifically, we represent a molecular system as a graph which contains a set of nodes, edges, faces etc. Interactions between these sets, which represent molecular subsystems in our case, are used to construct the potential energy surface for a reasonably sized chemical system with 51 dimensions. Essentially a family of neural networks that pertain to the graph-based subsystems, get the job done for this 51 dimensional system. We then ask if this same family of lower-dimensional neural networks can be transformed to provide accurate predictions for a 186 dimensional potential surface. We find that our algorithm does provide reasonably accurate results for this larger dimensional problem with sub-kcal/mol accuracy for the higher dimensional potential surface problem.
Via
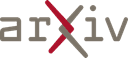
Nov 26, 2024
Abstract:Organic synthesis stands as a cornerstone of chemical industry. The development of robust machine learning models to support tasks associated with organic reactions is of significant interest. However, current methods rely on hand-crafted features or direct adaptations of model architectures from other domains, which lacks feasibility as data scales increase or overlook the rich chemical information inherent in reactions. To address these issues, this paper introduces {\modelname}, a novel chemical reaction representation learning model tailored for a variety of organic-reaction-related tasks. By integrating atomic correspondence between reactants and products, our model discerns the molecular transformations that occur during the reaction, thereby enhancing the comprehension of the reaction mechanism. We have designed an adapter structure to incorporate reaction conditions into the chemical reaction representation, allowing the model to handle diverse reaction conditions and adapt to various datasets and downstream tasks, e.g., reaction performance prediction. Additionally, we introduce a reaction-center aware attention mechanism that enables the model to concentrate on key functional groups, thereby generating potent representations for chemical reactions. Our model has been evaluated on a range of downstream tasks, including reaction condition prediction, reaction yield prediction, and reaction selectivity prediction. Experimental results indicate that our model markedly outperforms existing chemical reaction representation learning architectures across all tasks. Notably, our model significantly outperforms all the baselines with up to 25\% (top-1) and 16\% (top-10) increased accuracy over the strongest baseline on USPTO\_CONDITION dataset for reaction condition prediction. We plan to open-source the code contingent upon the acceptance of the paper.
Via
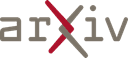
Nov 07, 2024
Abstract:Following the milestones in large language models (LLMs) and multimodal models, we have seen a surge in applying LLMs to biochemical tasks. Leveraging graph features and molecular text representations, LLMs can tackle various tasks, such as predicting chemical reaction outcomes and describing molecular properties. However, most current work overlooks the multi-level nature of graph features. The impact of different feature levels on LLMs and the importance of each level remain unexplored, and it is possible that different chemistry tasks require different feature levels. In this work, we first investigate the effect of feature granularity by fusing GNN-generated feature tokens, discovering that even reducing all tokens to a single token does not significantly impact performance. We then explore the effect of various feature levels on performance, finding that both the quality of LLM-generated molecules and performance on different tasks benefit from different feature levels. We conclude with two key insights: (1) current molecular Multimodal LLMs(MLLMs) lack a comprehensive understanding of graph features, and (2) static processing is not sufficient for hierarchical graph feature. Our code will be publicly available soon.
Via
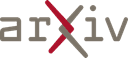
Nov 09, 2024
Abstract:The goal of this paper is to demonstrate and address challenges related to all aspects of performing a complete uncertainty quantification (UQ) analysis of a complicated physics-based simulation like a 2D slab burner direct numerical simulation (DNS). The UQ framework includes the development of data-driven surrogate models, propagation of parametric uncertainties to the fuel regression rate--the primary quantity of interest--and Bayesian calibration of critical parameters influencing the regression rate using experimental data. Specifically, the parameters calibrated include the latent heat of sublimation and a chemical reaction temperature exponent. Two surrogate models, a Gaussian Process (GP) and a Hierarchical Multiscale Surrogate (HMS) were constructed using an ensemble of 64 simulations generated via Latin Hypercube sampling. Both models exhibited comparable performance during cross-validation. However, the HMS was more stable due to its ability to handle multiscale effects, in contrast with the GP which was very sensitive to kernel choice. Analysis revealed that the surrogates do not accurately predict all spatial locations of the slab burner as-is. Subsequent Bayesian calibration of the physical parameters against experimental observations resulted in regression rate predictions that closer align with experimental observation in specific regions. This study highlights the importance of surrogate model selection and parameter calibration in quantifying uncertainty in predictions of fuel regression rates in complex combustion systems.
Via
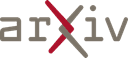
Sep 26, 2024
Abstract:Real-world chemical processes often exhibit stochastic dynamics with non-trivial correlations and state-dependent fluctuations. However, most process models simply add stationary noise terms to a deterministic prediction, which can lead to inaccurate predictions. This work proposes using conditional normalizing flows as discrete-time models (DTMs) to learn the stochastic dynamics of chemical processes. Normalizing flows learn an explicit expression of the system states' probability density function (PDF) given prior states and control inputs. The resulting model naturally allows for formulating stochastic and probabilistic setpoint-tracking objectives and chance constraints. In applications to a continuous reactor and a reactor cascade, the normalizing flow yields stable simulations over long time horizons and high-quality results in stochastic and probabilistic MPC formulation for open-loop control. Furthermore, a chance-constrained optimization finds reliable startup controls for the reactor cascade with stochastic reactions. In conclusion, the conditional normalizing flow presents an excellent choice for modeling nonlinear stochastic dynamics.
* 13 pages, 7 Figures, 5 Tables
Via
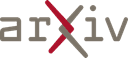
Aug 19, 2024
Abstract:Retrosynthesis analysis is pivotal yet challenging in drug discovery and organic chemistry. Despite the proliferation of computational tools over the past decade, AI-based systems often fall short in generalizing across diverse reaction types and exploring alternative synthetic pathways. This paper presents BatGPT-Chem, a large language model with 15 billion parameters, tailored for enhanced retrosynthesis prediction. Integrating chemical tasks via a unified framework of natural language and SMILES notation, this approach synthesizes extensive instructional data from an expansive chemical database. Employing both autoregressive and bidirectional training techniques across over one hundred million instances, BatGPT-Chem captures a broad spectrum of chemical knowledge, enabling precise prediction of reaction conditions and exhibiting strong zero-shot capabilities. Superior to existing AI methods, our model demonstrates significant advancements in generating effective strategies for complex molecules, as validated by stringent benchmark tests. BatGPT-Chem not only boosts the efficiency and creativity of retrosynthetic analysis but also establishes a new standard for computational tools in synthetic design. This development empowers chemists to adeptly address the synthesis of novel compounds, potentially expediting the innovation cycle in drug manufacturing and materials science. We release our trial platform at \url{https://www.batgpt.net/dapp/chem}.
Via
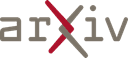
Jul 21, 2024
Abstract:High-throughput reaction condition (RC) screening is fundamental to chemical synthesis. However, current RC screening suffers from laborious and costly trial-and-error workflows. Traditional computer-aided synthesis planning (CASP) tools fail to find suitable RCs due to data sparsity and inadequate reaction representations. Nowadays, large language models (LLMs) are capable of tackling chemistry-related problems, such as molecule design, and chemical logic Q\&A tasks. However, LLMs have not yet achieved accurate predictions of chemical reaction conditions. Here, we present MM-RCR, a text-augmented multimodal LLM that learns a unified reaction representation from SMILES, reaction graphs, and textual corpus for chemical reaction recommendation (RCR). To train MM-RCR, we construct 1.2 million pair-wised Q\&A instruction datasets. Our experimental results demonstrate that MM-RCR achieves state-of-the-art performance on two open benchmark datasets and exhibits strong generalization capabilities on out-of-domain (OOD) and High-Throughput Experimentation (HTE) datasets. MM-RCR has the potential to accelerate high-throughput condition screening in chemical synthesis.
Via
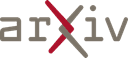